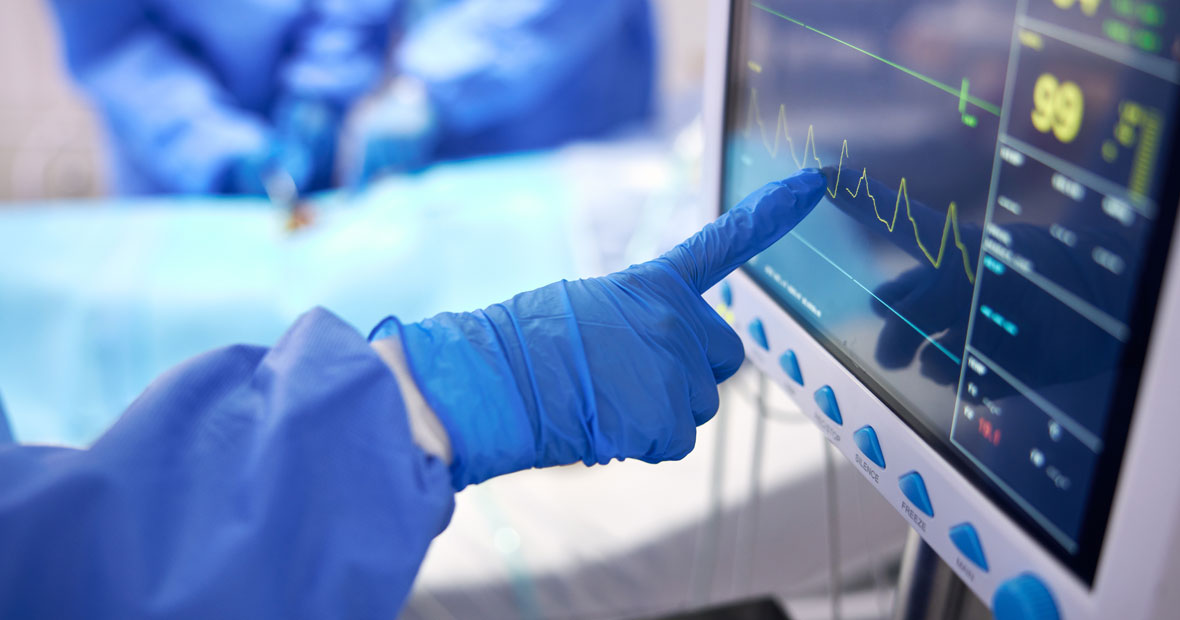
Delving into the realm of model monitoring tools, this piece takes a comprehensive look at their role in enhancing performance and reliability. From key features that make these tools effective, to the significance of real-time data tracking, security considerations, and the impact of community support, every facet is meticulously examined. The discussion further extends to MLOps tools, their part in boosting accuracy, the role of data quality, early issue identification, and the importance of continuous learning. The journey culminates with insights on maintaining model quality from training to production. A wealth of knowledge awaits in the subsequent text, offering an enlightening perspective on these indispensable assets in the technological landscape.
Key Features of Effective Model Monitoring Tools
Effective model monitoring tools serve as a pivotal resource for businesses, offering the capability to swiftly detect issues, maintain model accuracy, and enhance overall performance. The proper use of these tools plays a critical role in identifying model errors before they escalate into substantial problems.
Significance of Real-time Data Tracking in Monitoring Tools
Model monitoring tools come in various types, each with unique features that set them apart. A key attribute, however, is the ability to track real-time data. This feature enables businesses to monitor performance metrics and identify issues as they arise. The detection prowess of these tools aids in making data-driven decisions and enhances forecast accuracy. This real-time analysis further optimizes business processes, thereby boosting productivity.
Security Aspects to Consider in Model Monitoring Tools
Model monitoring tools not only help in maintaining model quality but also assist in complying with regulations. Security is paramount, and these tools help businesses navigate potential risks by detecting fairness and bias issues in the models. Addressing such issues is vital in maintaining the integrity of the models, thereby ensuring business continuity.
Role of Community Support in Enhancing Tool Efficiency
With model monitoring tools, collaboration across different departments within the organization becomes effortless. The tools are easy to integrate with other technologies, thereby fostering a seamless working environment. Community support further enhances tool efficiency by offering solutions to common challenges and facilitating knowledge sharing. This collaborative approach helps automate the model maintenance process, adding to the overall efficiency of the organization.
Boosting Accuracy and Reliability with MLOps Tools
Within the sphere of machine learning operations, the quest for improved accuracy and reliability continues. The right MLOps tools can significantly enhance the performance of machine learning models.
Role of Data Quality in Enhancing Model Accuracy
The quality of data employed plays a pivotal role in boosting model accuracy. MLOps tools support this by ensuring the data used is of optimal quality, effectively enhancing the contribution of machine learning to the business. These tools also provide a platform for open community-based learning, fostering a network of knowledge-sharing and innovation.
Identifying Issues Early with MLOps Tool Metrics
MLOps tools offer an array of metrics designed to identify issues early on, supporting the swift resolution of potential problems. By utilizing this software, businesses can monitor the reliability of their machine learning models, ensuring they function at their peak. These metrics are sourced from trusted and reliable academic and educational sites, scientific publications, government sites, international organizations, and research institutes.
Importance of Continuous Learning in MLOps Tools
Continuous learning remains a cornerstone within the realm of MLOps. These tools provide opportunities for ongoing learning, thereby enhancing the accuracy and reliability of machine learning models. Businesses can leverage these resources to drive innovation and improve overall performance. The security of data used during this learning process is paramount, and MLOps tools have measures in place to safeguard this.
From Training to Production: Ensuring Model Quality Over Time
Keeping a consistent quality in models during the shift from training to production poses a considerable challenge. Yet, the right strategies and techniques provide the necessary assistance to overcome these difficulties. The use of effective model monitoring tools significantly enhances the performance and reliability of data models. These tools play a pivotal role in identifying and handling model drift, a common issue that arises due to changes in the underlying data.
Model drift, essentially, refers to the discrepancy between the predictions made by the model during training and the actual outcome in real-time situations. This drift, if not addressed promptly, can lead to significant performance degradation. Hence, dealing with model drift needs a proactive approach and a robust set of tools to track and manage changes in data patterns.
Quality assurance in production also involves effectively managing real-time data predictions. This involves dealing with new input data or different data, which requires an adaptive system. Solutions to these issues help maintain the quality of the models over time, thus ensuring reliable performance. By adopting these strategies, the transition from training to production can be managed more effectively, thereby maintaining the quality and reliability of the models in the long run.